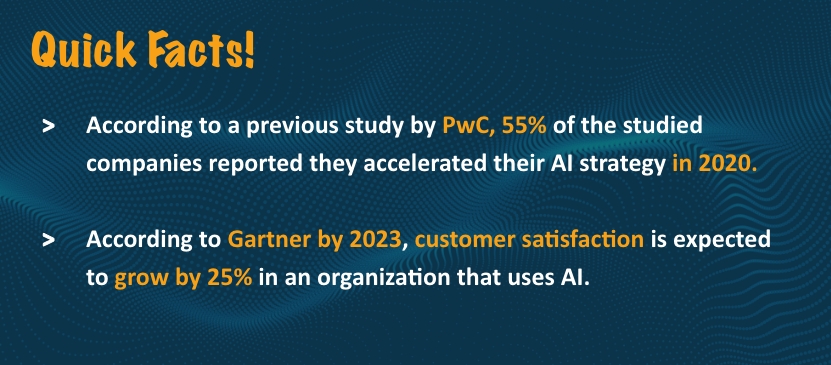
Constant technology innovations have eased the overall pain of building a business. People can now leverage the power of technology to scale their businesses. However, at the same time, it has become difficult and perhaps inevitable to gain a competitive advantage in your field of work. One sure-short way of leading a business to failure is lacking innovation and not staying ahead of the competition.
Nevertheless, there's good news! Businesses can now leverage the tremendous power of AI undefined Machine learning to stay ahead of the competition by generating key AI-ML-based business intelligence insights.
Whether you’re using AI-ML for business intelligence or for automating your businesses, you are way ahead of your competition because you’re making your data work for you!
In this blog, we focus on predictive business intelligence using machine learning, in simple terms, machine learning forecasting as a catalyst for business growth.
Making business-generated data work for you is possibly the wisest decision a business can make. Business forecasting guides a business into the future with better and more advanced decision-making methods than traditional ones. ML-backed forecasting helps businesses to predict and deal with any possible issue beforehand, be it a logistical issue, running out of stock, or even minimizing loss functions, machine learning forecasting got it all covered for you!
1. Competitive Advantage: Predicting crucial business parameters, which would have not been possible using traditional methods, gives businesses an exceptional competitive advantage. | ||
2. Fewer Errors: ML-backed forecasting reduced the number of logistical errors in supply chain-intensive businesses. ML algorithms help businesses to make the best logistical decisions and serve their customers the best. | ||
3. High Adaptability: ML forecasting algorithms can quickly adapt to changes, while still maintaining accuracy. | ||
4. Self-Growth: Ability to make ML models more accurate and precise by feeding more relevant data over a period of time. Thus, better predictions for business. |
Supply chain undefined logistics forecastingML-based predictions are steadily becoming an integral part of the supply chain management processes. It truly helps to streamline the whole supply chain with effective management of stocks. Understocking and overstocking can be avoided with customer requirement forecasting with Just-in-time idealogy, which helps to cut costs at the right places. ML-based forecasting would let businesses be at par with inventory management and product supply. Thus, providing the best customer service at a cost-effective rate. |
Financial forecastingOne of the primary challenges, especially for FMCGs and product-led businesses, is the constant change in demand. The inability to forecast demand changes and not cope with them can lead to customer dissatisfaction and business loss. ML-based forecasting helps business owners with future demand prediction and avoids inventory overloading or underloading by having just the right amount of inventory needed. |
Demand forecastingOne of the primary challenges, especially for FMCGs and product-led businesses, is the constant change in demand. The inability to forecast demand changes and not cope with them can lead to customer dissatisfaction and business loss. ML-based forecasting helps business owners with future demand prediction and avoids inventory overloading or underloading by having just the right amount of inventory needed. |
As the world moves towards better and more advanced technology practices, it becomes crucial for companies to adapt to the change and use advanced technologies to improve their businesses.
When it comes to running a business and scaling it, a competitive edge is what sets successful companies apart: staying ahead of the competition, forecasting business and customer needs beforehand and serving the customer in the best possible manner.
We, at Seaflux, are AI undefined Machine Learning enthusiasts, who are helping enterprises worldwide. Have a query or want to discuss AI projects where the cloud can be leveraged? Schedule a meeting with us here, we'll be happy to talk to you.
Director of Engineering