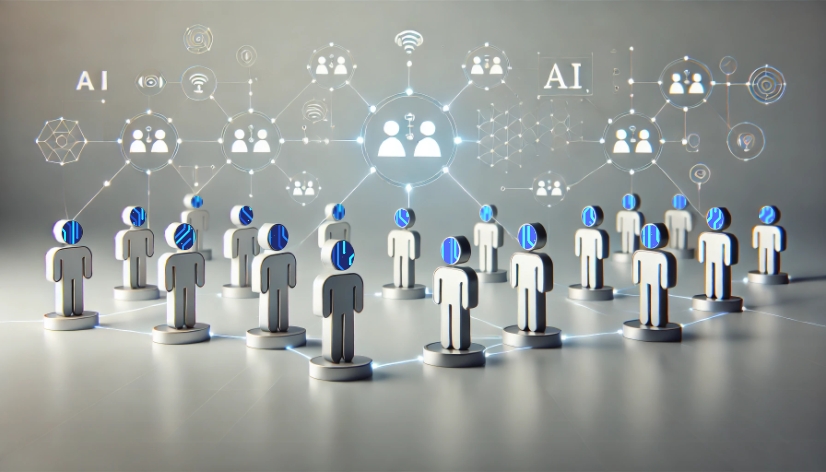
AI has been advancing at a very rapid pace in the technology landscape. Businesses started with basic mechanical intervention in the 90s to increase efficiency, and are now leveraging AI to automate their business operations as a whole. This automation has led to the rise of businesses relying on AI and branching a new way of automation - AI Agents. AI Agents are independent task managers who are assigned a specific task, and it performs automatically. AI agents use the latest - Gen AI and NLP capabilities in order to complete the tasks.
In this blog, let's understand:
We will also look at 2 potential cases that businesses need to know and how an organization should prepare for future adaptation.
The base of AI Agents is Perceive undefined Analyze undefined Learn undefined Act undefined Repeat, mimicking human-like behavior and decision-making capabilities. AI Agents are intelligent independent systems, capable of perceiving and learning from their environment as well as experience/feedback, and take actions that help them achieve specific goals. These goals can be assigned and they work to achieve them and keep upgrading themselves with every interaction. A very go-to example can be an autonomous car driving solution. It may sound like a simple task, however, it's a much more complex task to develop one.
An AI Agent can range from offering a simplistic reflex-based or sophisticated learning-based decision-making. Each type has its own set of features that can be useful according to the requirement. Let us delve into each type of AI Agents:
Use case: A simple robot reacting to the real-time environment condition only with the trained data.
AI Agents learn from the data either provided or acquired from the environment and make decisions based on the predefined rules and gathered knowledge. Here's how an ideal AI Agent would work using AI several capabilities:
Perception is the gathering of information from the real world through the sensors or inputs, such as cameras, microphones, or data feeds. The data is then translated into a format that the agent can understand. For example, a chatbot would use a microphone to collect a user's voice and convert it into text that the agent would understand.
Agents learn from experience, either through supervised learning (labeled data), unsupervised learning (unlabeled data), or reinforcement learning (trial and error). This enables agents to adapt to new situations, improve their performance, and acquire new knowledge and skills. For instance, a machine translation agent might learn to translate text from one language to another by analyzing millions of pairs of translated sentences (supervised learning). A self-driving car might learn to navigate roads by driving in a variety of conditions and receiving feedback on its performance (reinforcement learning).
After learning from the environment or data, the agents would analyze information, identify the patterns, and make inferences about the present situation. Considering all the aspects, the agent would then make a decision, solve a problem, or derive a plan of action to proceed with the solution. For example, a chess-playing agent would use its chess rules and strategies based on the opponent's moves to evaluate different paths and choose the best one.
While assigning the objectives, the agents are authorized to act by responding or providing solutions in the environment such as controlling devices, human interaction, or making movement. This is the output from the agent after executing the plan to achieve the specified goal. For example, a robot moves its arms to maneuver objects, while a chatbot responds to user queries with relevant information.
The process of perception, learning, reasoning, and action is a continuous cycle. After the completion of the Action, the AI agent would gather the feedback of its outcome and treat that as the perception to improve their knowledge as well as behavior and hence the outcome in the next interaction. This adapts the agents to dynamic environments and improves their performance over time.
AI agents can offer significant advantages to businesses across various industries. AI agents are capable of perceiving their environment, learning from experience, and taking actions to achieve specific goals, offer several advantages over traditional AI approaches:
The capability of AI agents to be designed with specific goals enables them to proactively seek out information or opportunities to achieve those objectives. On the other hand, traditional AI systems would require explicit instructions or queries.
AI agents interact with their environment to learn and adjust their behavior based on rewards or punishments. This reinforcement learning allows them to adapt according to the changing scenarios and improve their performance over time.
As the AI agents interact with their environment, they perceive the information and make decisions in real-time based on the present scenario, enabling them to respond quickly to dynamic changes.
When embodied in a physical system such as robots, AI agents can interact with the real world and perform tasks that traditional AI systems might struggle with.
AI agents have the capability to operate with each other in a synergy to accomplish a large-scale or complex problem. Traditional AI often operates in isolation or requires centralized control.
Businesses can leverage these agents to increase operational efficiency and offer unparalleled customer service. Let us understand the impact on the business using two AI agent applications where the Agents can be deployed.
Opportunity: Financial institutions tend to carry out a credit risk analysis of the borrower before lending a credit or loan based on certain information like payment history, types of loans, and other factors. With the variety of credit risks, an assigned relationship manager needs to work with the borrower, credit analysts, and all the stakeholders multiple times to prepare a credit report and pass it on to the credit manager for review and further analysis before loan disbursement. This is a time-consuming process and requires a lot of man hours to complete the task.
AI Agent Solution: Deploying multiple AI Agents at each level with specific goals would decrease the amount of time required to complete the process and provide a much more accurate credit report without any human intervention. The report will then be sent to the credit manager for final review and provide the expertise for loan authorization and disbursement.
Opportunity: The FMCG industry performs a lot on the Just In Time (JIT) strategy. This helps the businesses keep their cost in check and offer the best quality of the products. However, keeping the stocks at the retail end can be a task and the strategy needs to be perfect to avoid the disruption of the product availability. The supply chain plays a vital role, where the time taken for each task from packaging to shipment and actual delivery must be in synergy to keep the stocks on the shelf.
AI Agent Solution: A set of multiple AI agents can be deployed where the agents would keep an eye on the remaining stocks of the goods, predict future sales, and communicate the required number of goods to the supplier side agent well in advance. The supplier agent would place the required number of orders and manage the stock from all the available vendors. An AI agent would predict the road conditions, weather forecast, and other factors impacting the time taken for the delivery and set the date of shipment such as the stock reaching the shelves on time.
AI Agents are micro intelligent agents performing individually to complete their tasks and achieve the overall goal. Each AI agent has its own importance and is required to complete the task. We have gone through what AI agents are, the types of AI agents, and the benefits of AI agents. We have also understood how AI agents work and to support that we dig into two AI agents' use cases.
We, at Seaflux, are AI undefined Machine Learning enthusiasts, who are helping enterprises worldwide. Have a query or want to discuss AI projects where LiteLLM can be leveraged? Schedule a meeting with us here, we'll be happy to talk to you.
Senior Marketing Executive